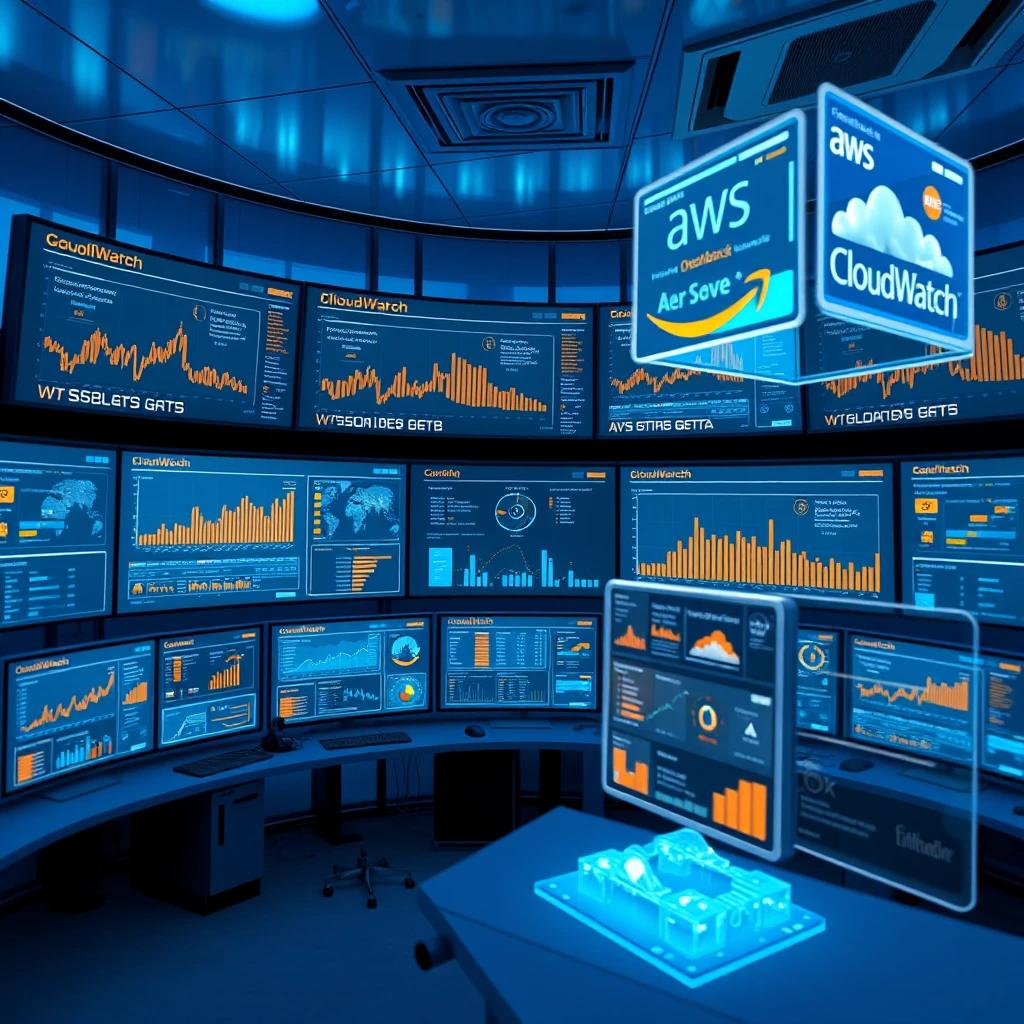
Suppose you’re constructing a complex house. You wouldn’t just glance at the front door to check if everything is fine, you’d inspect the foundation, wiring, plumbing, and how everything connects. Modern cloud applications demand the same thoroughness, and AWS CloudWatch acts as your sophisticated inspector. In this article, let’s explore some advanced features of CloudWatch that often go unnoticed but can transform your cloud observability.
The art of smart alerting with composite alarms
Think back to playing with building blocks as a kid. You could stack them to build intricate structures. CloudWatch’s composite alarms work the same way. Instead of triggering an alarm every time one metric exceeds a threshold, you can combine multiple conditions to create smarter, context-aware alerts.
For instance, in a critical web application, high CPU usage alone might not indicate an issue, it could just be handling a traffic spike. But combine high CPU with increasing error rates and declining response times, and you’ve got a red flag. Here’s an example:
CompositeAlarm:
- Condition: CPU Usage > 80% for 5 minutes
AND
- Condition: Error Rate > 1% for 3 minutes
AND
- Condition: Response Time > 500ms for 3 minutes
Take this a step further with Anomaly Detection. Instead of rigid thresholds, Anomaly Detection learns your system’s normal behavior patterns and adjusts dynamically. It’s like having an experienced operator who knows what’s normal at different times of the day or week. You select a metric, enable Anomaly Detection, and configure the expected range based on historical data to enable this.
Exploring Step Functions and CloudWatch Insights
Now, let’s dive into a less-discussed yet powerful feature: monitoring AWS Step Functions. Think of Step Functions as a recipe, each step must execute in the right order. But how do you ensure every step is performing as intended?
CloudWatch provides detective-level insights into Step Functions workflows:
- Tracing State Flows: Each state transition is logged, letting you see what happened and when.
- Identifying Bottlenecks: Use CloudWatch Logs Insights to query logs and find steps that consistently take too long.
- Smart Alerting: Set alarms for patterns, like repeated state failures.
Here’s a sample query to analyze Step Functions performance:
fields @timestamp, @message
| filter type = "TaskStateEntered"
| stats avg(duration) as avg_duration by stateName
| sort by avg_duration desc
| limit 5
Armed with this information, you can optimize workflows, addressing bottlenecks before they impact users.
Managing costs with CloudWatch optimization
Let’s face it, unexpected cloud bills are never fun. While CloudWatch is powerful, it can be expensive if misused. Here are some strategies to optimize costs:
1. Smart metric collection
Categorize metrics by importance:
- Critical metrics: Collect at 1-minute intervals.
- Important metrics: Use 5-minute intervals.
- Nice-to-have metrics: Collect every 15 minutes.
This approach can significantly lower costs without compromising critical insights.
2. Log retention policies
Treat logs like your photo library: keep only what’s valuable. For instance:
- Security logs: Retain for 1 year.
- Application logs: Retain for 3 months.
- Debug logs: Retain for 1 week.
Set these policies in CloudWatch Log Groups to automatically delete old data.
3. Metric filter optimization
Avoid creating a separate metric for every log event. Use metric filters to extract multiple insights from a single log entry, such as response times, error rates, and request counts.
Exploring new frontiers with Container Insights and Cross-Account Monitoring
Container Insights
If you’re using containers, Container Insights provides deep visibility into your containerized environments. What makes this stand out? You can correlate application-specific metrics with infrastructure metrics.
For example, track how application error rates relate to container restarts or memory spikes:
MetricFilters:
ApplicationErrors:
Pattern: "ERROR"
Correlation:
- ContainerRestarts
- MemoryUtilization
Cross-Account monitoring
Managing multiple AWS accounts can be a complex challenge, especially when trying to maintain a consistent monitoring strategy. Cross-account monitoring in CloudWatch simplifies this by allowing you to centralize your metrics, logs, and alarms into a single monitoring account. This setup provides a “single pane of glass” view of your AWS infrastructure, making it easier to detect issues and streamline troubleshooting.
How it works:
- Centralized Monitoring Account: Designate one account as your primary monitoring hub.
- Sharing Metrics and Dashboards: Use AWS Resource Access Manager (RAM) to share CloudWatch data, such as metrics and dashboards, between accounts.
- Cross-Account Alarms: Set up alarms that monitor metrics from multiple accounts, ensuring you’re alerted to critical issues regardless of where they occur.
Example: Imagine an organization with separate accounts for development, staging, and production environments. Each account collects its own CloudWatch data. By consolidating this information into a single account, operations teams can:
- Quickly identify performance issues affecting the production environment.
- Correlate anomalies across environments, such as a sudden spike in API Gateway errors during a new staging deployment.
- Maintain unified dashboards for senior management, showcasing overall system health and performance.
Centralized monitoring not only improves operational efficiency but also strengthens your governance practices, ensuring that monitoring standards are consistently applied across all accounts. For large organizations, this approach can significantly reduce the time and effort required to investigate and resolve incidents.
How CloudWatch ServiceLens provides deep insights
Finally, let’s talk about ServiceLens, a feature that integrates CloudWatch with X-Ray traces. Think of it as X-ray vision for your applications. It doesn’t just tell you a request was slow, it pinpoints where the delay occurred, whether in the database, an API, or elsewhere.
Here’s how it works: ServiceLens combines traces, metrics, and logs into a unified view, allowing you to correlate performance issues across different components of your application. For example, if a user reports slow response times, you can use ServiceLens to trace the request’s path through your infrastructure, identifying whether the issue stems from a database query, an overloaded Lambda function, or a misconfigured API Gateway.
Example: Imagine you’re running an e-commerce platform. During a sale event, users start experiencing checkout delays. Using ServiceLens, you quickly notice that the delay correlates with a spike in requests to your payment API. Digging deeper with X-Ray traces, you discover a bottleneck in a specific DynamoDB query. Armed with this insight, you can optimize the query or increase the DynamoDB capacity to resolve the issue.
This level of integration not only helps you diagnose problems faster but also ensures that your monitoring setup evolves with the complexity of your cloud applications. By proactively addressing these bottlenecks, you can maintain a seamless user experience even under high demand.
Takeaways
AWS CloudWatch is more than a monitoring tool, it’s a robust observability platform designed to meet the growing complexity of modern applications. By leveraging its advanced features like composite alarms, anomaly detection, and ServiceLens, you can build intelligent alerting systems, streamline workflows, and maintain tighter control over costs.
A key to success is aligning your monitoring strategy with your application’s specific needs. Rather than tracking every metric, focus on those that directly impact performance and user experience. Start small, prioritizing essential metrics and alerts, then incrementally expand to incorporate advanced features as your application grows in scale and complexity.
For example, composite alarms can reduce alert fatigue by correlating multiple conditions, while ServiceLens provides unparalleled insights into distributed applications by unifying traces, logs, and metrics. Combining these tools can transform how your team responds to incidents, enabling faster resolution and proactive optimization.
With the right approach, CloudWatch not only helps you prevent costly outages but also supports long-term improvements in your application’s reliability and cost efficiency. Take the time to explore its capabilities and tailor them to your needs, ensuring that surprises are kept at bay while your systems thrive.