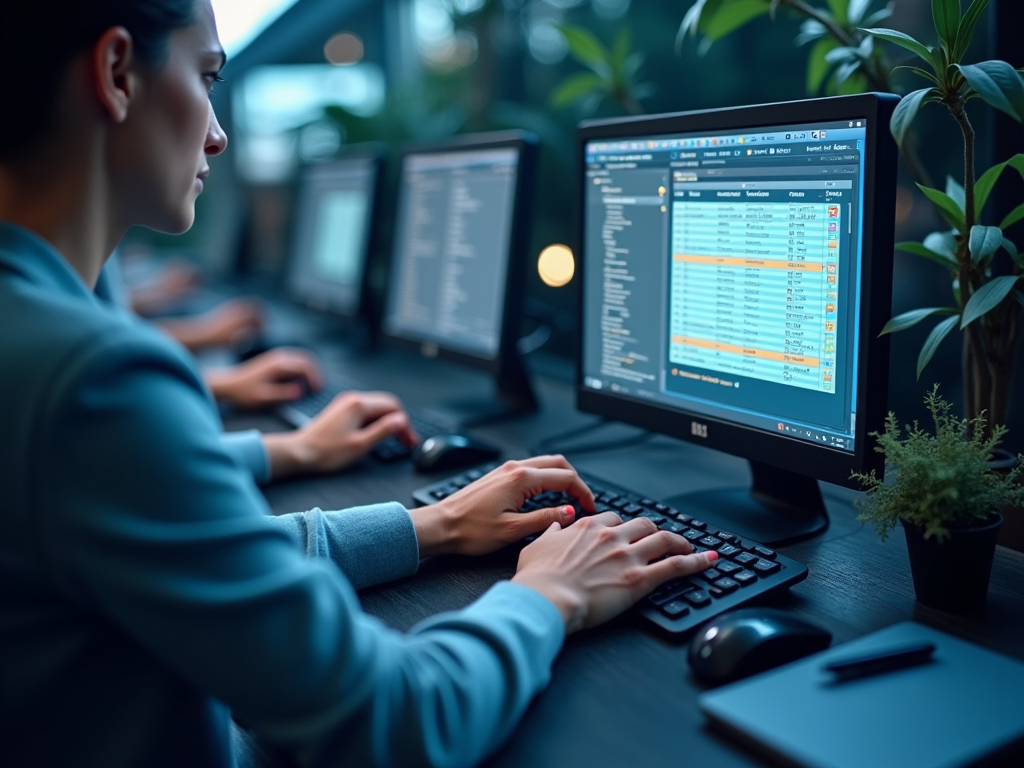
Picture yourself running a restaurant. Every morning before opening, you would check different things: Are the refrigerators working? Is there power in the building? Does the kitchen equipment function properly? These checks ensure your restaurant can serve customers effectively. Similarly, Amazon Web Services (AWS) performs various checks on your EC2 instances to ensure they’re running smoothly. Let’s break this down in simple terms.
What are EC2 status checks?
Think of EC2 status checks as your instance’s health monitoring system. Just like a doctor checks your heart rate, blood pressure, and temperature, AWS continuously monitors different aspects of your EC2 instances. These checks happen automatically every minute, and best of all, they are free!
The three types of status checks
1. System status checks as the building inspector
System status checks are like a building inspector. They focus on the infrastructure rather than what is happening in your instance. These checks monitor:
- The physical server’s power supply
- Network connectivity
- System software
- Hardware components
When a system status check fails, it is usually an issue outside your control. It is akin to when your apartment building loses power – there’s not much you can do personally to fix it. In these cases, AWS is responsible for the repairs.
What can you do if it fails?
- Wait for AWS to fix the underlying problem (similar to waiting for the power company to restore electricity).
- You can move your instance to a new “building” by stopping and starting it (note: this is different from simply rebooting).
2. Instance status checks as your personal space monitor
Instance status checks are like having a smart home system that monitors what is happening inside your apartment. These checks look at:
- Your instance’s operating system
- Network configuration
- Software settings
- Memory usage
- File system status
- Kernel compatibility
When these checks fail, it typically means there’s an issue you need to address. It is similar to accidentally tripping a circuit breaker in your apartment – the infrastructure is fine, but the problem is within your own space.
How to fix instance status check failures:
- Restart your instance (like resetting that tripped circuit breaker).
- Review and modify your instance configuration.
- Make sure your instance has enough memory.
- Check for corrupted file systems and repair them if needed.
3. EBS status checks as your storage guardian
EBS status checks are like monitoring your external storage unit. They monitor the health of your attached storage volumes and can detect issues like:
- Hardware problems with the storage system
- Connectivity problems between your instance and its storage
- Physical host issues affecting storage access
What to do if EBS checks fail:
- Restart your instance to try to restore connectivity.
- Replace problematic EBS volumes.
- Check and fix any connectivity issues.
How to monitor these checks
Monitoring status checks is straightforward, and you have several options:
- Using the AWS management console
- Open the EC2 console.
- Select your instance.
- Look at the “Status Checks” tab.
It’s that simple! You’ll see either a green check (passing) or a red X (failing) for each type of check.
Setting up automated monitoring
Now, here’s where things get interesting. You can set up Amazon CloudWatch to alert you if something goes wrong. It is like having a security system that notifies you if there is an issue.
Here’s a simple example:
aws cloudwatch put-metric-alarm \
--alarm-name "Instance-Health-Check" \
--namespace "AWS/EC2" \
--metric-name "StatusCheckFailed" \
--dimensions Name=InstanceId,Value=i-1234567890abcdef0 \
--period 300 \
--evaluation-periods 2 \
--threshold 1 \
--comparison-operator GreaterThanOrEqualToThreshold \
--alarm-actions arn:aws:sns:region:account-id:topic-name
Each parameter here has its purpose:
- –alarm-name: The name of your alarm.
- –namespace and –metric-name: These identify the CloudWatch metric you are interested in.
- –dimensions: Specifies the instance ID being monitored.
- –period and –evaluation-periods: Define how often to check and for how long.
- –threshold and –comparison-operator: Set the condition for triggering an alarm.
- –alarm-actions: The action to take if the alarm state is triggered, like notifying you via SNS.
You could also set up these alarms through the AWS Management Console, which offers an intuitive UI for configuring CloudWatch.
Best practices for status checks
1. Don’t wait for problems
- Set up CloudWatch alarms for all critical instances.
- Monitor trends in status check results.
- Document common issues and their solutions to improve response times.
2. Automate recovery
- Configure automatic recovery actions for system status check failures.
- Create automated backup systems and recovery procedures.
- Test recovery processes regularly to ensure they work when needed.
3. Keep records
- Log all status check failures.
- Document steps taken to resolve issues.
- Track recurring problems and implement solutions to prevent future failures.
Cost considerations
The good news? Status checks themselves are free! However, some recovery actions might incur costs, such as:
- Starting and stopping instances (which might change your public IP).
- Data transfer costs during recovery.
- Additional EBS volumes if replacements are needed.
Real-World example
Imagine you receive an alert at 3 AM about a failed system status check. Here is how you might handle it:
- Check the AWS status page: See if there is a broader AWS issue.
- If it is isolated to your instance:
- Stop and start the instance (not just reboot).
- Check if the issue persists once the instance moves to new hardware.
- If the problem continues:
- Review instance logs for more clues.
- Contact AWS Support if the issue is beyond your expertise or remains unresolved.
Final thoughts
EC2 status checks are your early warning system for potential problems. They are simple to understand but incredibly powerful for keeping your applications running smoothly. By monitoring these checks and setting up appropriate alerts, you can catch and address problems before they impact your users.
Remember: the best problems are the ones you prevent, not the ones you fix. Regular monitoring and proper setup of status checks will help you sleep better at night, knowing your instances are being watched over.
Next time you log into your AWS console, take a moment to check your status checks. They’re like a 24/7 health monitoring system for your cloud infrastructure, ensuring you maintain a healthy, reliable system.