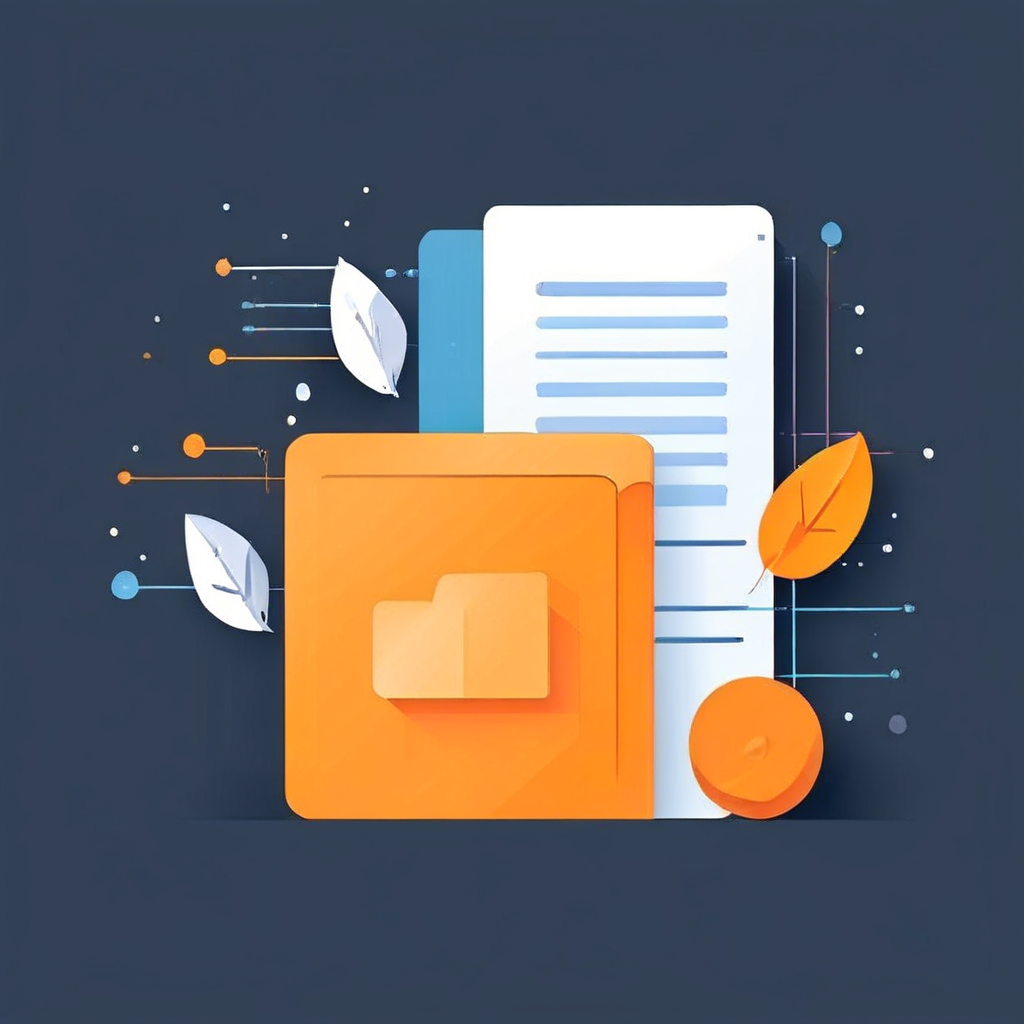
When we talk about caching in AWS, we’re referring to a variety of strategies that improve the performance and efficiency of your applications. Caching is a powerful tool that helps in reducing latency, offloading demand from the primary data source, and enhancing user experience. In this article, we’ll explore four primary AWS caching solutions: Amazon CloudFront, Amazon EC2 in-memory caches, Amazon ElastiCache, DynamoDB Accelerator (DAX) and session caching.
Let’s dive in and understand each one in a way that’s straightforward to grasp.
1. Amazon CloudFront: Speeding Up Content Delivery
Imagine you have a website with lots of images, videos, and other static files. Every time someone visits your site, these files must be loaded, which can take time, especially if your visitors are spread around the globe. This is where Amazon CloudFront comes in.
Amazon CloudFront is a Content Delivery Network (CDN). Think of it as a network of servers strategically placed around the world. When a user requests content from your website, CloudFront delivers it from the nearest server location, called an edge location. This significantly speeds up content delivery, improving user experience.
Here’s a common setup:
- Store your static files (like HTML, CSS, JavaScript, and images) in an Amazon S3 bucket.
- Create a CloudFront distribution linked to your S3 bucket.
- Deploy your content to edge locations globally.
When a user accesses your site, CloudFront fetches the content from the nearest edge location, ensuring quick and efficient delivery.
2. Amazon EC2 In-Memory Caching: Quick Data Access
For dynamic content and frequently accessed data, in-memory caching can be a game-changer. Amazon EC2 allows you to set up a local cache directly in the memory of your virtual machine.
In-memory caches store data in RAM, making data retrieval incredibly fast. Here’s how it works:
- Suppose you’re using a Java application. You can leverage frameworks like Guava to cache data in the EC2 instance’s memory.
- This means that instead of repeatedly fetching data from a database, your application can quickly access it from the local cache.
However, there’s a caveat. If your EC2 instance is restarted or terminated, the cached data is lost. This is where the need for a more persistent caching solution might arise.
3. Amazon ElastiCache: Scalable and Reliable Caching
For a robust and distributed caching solution, Amazon ElastiCache is your go-to service. ElastiCache supports two popular caching engines: Redis and Memcached.
- Redis is renowned for its rich set of features including support for complex data structures like lists, sets, and sorted sets. It’s versatile and widely used, offering capabilities beyond simple caching.
- Memcached is simpler, focusing on high-performance and easy-to-use caching of key-value pairs. It’s multi-threaded, which can result in better performance in some scenarios.
ElastiCache operates outside your compute infrastructure, meaning it’s not tied to any single EC2 instance. This makes it a reliable option for maintaining cache continuity even if your application servers change.
4. DynamoDB Accelerator (DAX): Turbocharging NoSQL
When using Amazon DynamoDB for its scalable NoSQL capabilities, you might find that you need even faster read performance. This is where DynamoDB Accelerator (DAX) comes into play.
DAX is an in-memory caching service specifically designed for DynamoDB. It can reduce read latency from milliseconds to microseconds by caching the frequently accessed data. Setting up DAX is straightforward:
- Attach DAX to your existing DynamoDB tables.
- Configure your application to use DAX for read and write operations.
DAX is handy for read-heavy applications where quick data retrieval is critical.
5. Session Caching: Managing User Sessions Efficiently
In web applications, managing user session data efficiently is crucial for performance and user experience. Storing session data in a database can lead to high latency and increased load on the database, especially for applications with heavy traffic. This is where ElastiCache comes to the rescue with its ability to handle session caching.
ElastiCache can store session data in memory, providing a faster and more scalable alternative to database storage. Here’s how it works:
- Session data (like user login information, preferences, and temporary data) is stored in an ElastiCache cluster.
- Redis is often the preferred choice for session caching due to its support for complex data structures and persistence options.
- Memcached can also be used if you need a simple key-value store with high performance.
By using ElastiCache for session caching, your application can:
- Reduce latency: Retrieve session data quickly from memory instead of querying a database.
- Scale seamlessly: Handle high traffic volumes without impacting database performance.
- Ensure reliability: Use features like Redis’ replication and failover mechanisms to maintain session data availability.
Implementing session caching with ElastiCache can significantly enhance the performance and scalability of your web applications, providing a smoother experience for your users.
Effective Caching in AWS
Understanding these caching solutions can greatly enhance your AWS architecture. Whether you’re accelerating static content delivery with CloudFront, boosting dynamic data access with EC2 in-memory caches, implementing a robust and scalable cache with ElastiCache, speeding up your DynamoDB operations with DAX, or managing user sessions efficiently, each solution serves a unique purpose.
Remember, the goal of caching is to reduce latency and improve performance. By leveraging these AWS services effectively, we can ensure our applications are faster, more responsive, and able to handle higher loads efficiently.