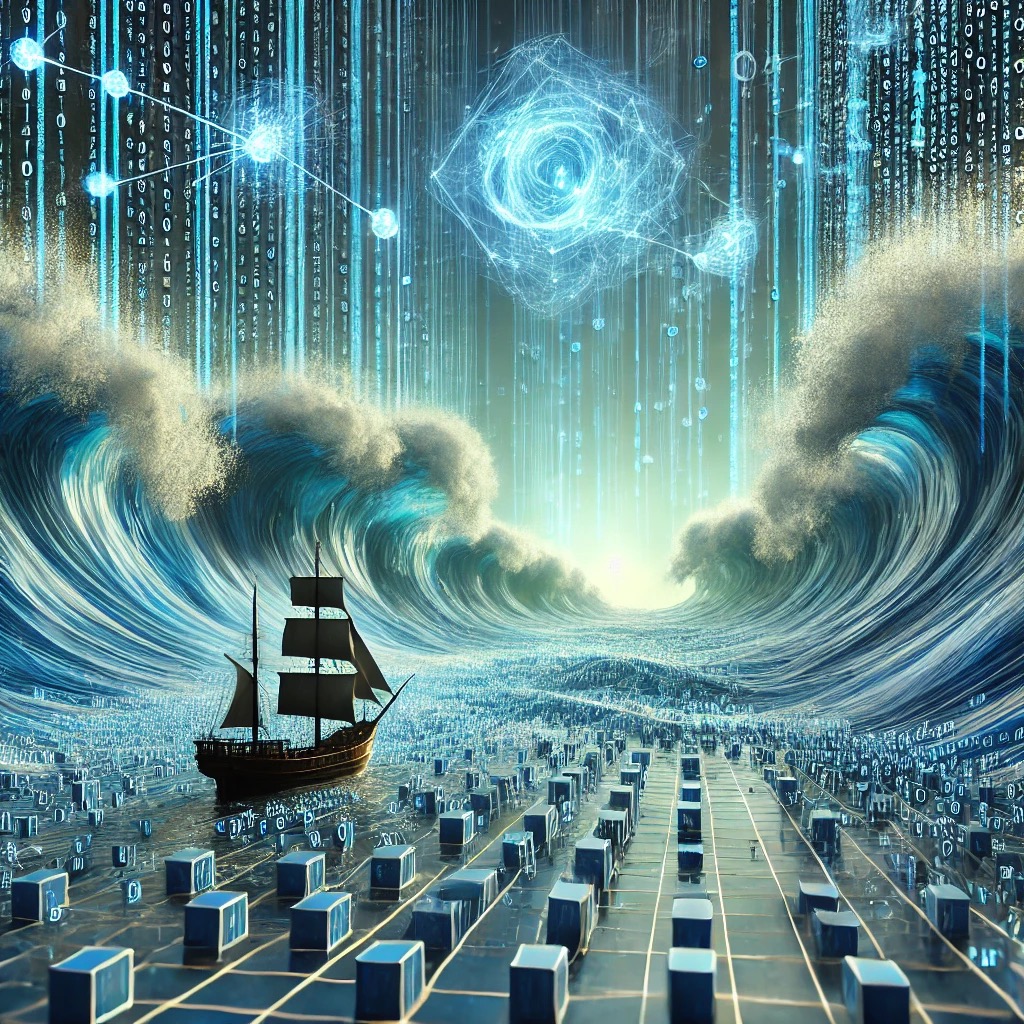
Imagine this: you’re a seasoned sailor, a master of the seas, confident in navigating any storm. But suddenly, the ocean beneath your ship becomes a swirling vortex of unpredictable currents and shifting waves. Welcome to Site Reliability Engineering (SRE) in the age of Generative AI.
The shifting tides of SRE
For years, SREs have been the unsung heroes of the tech world, ensuring digital infrastructure runs as smoothly as a well-oiled machine. They’ve refined their expertise around automation, monitoring, and observability principles. But just when they thought they had it all figured out, Generative AI arrived, turning traditional practices into a tsunami of new challenges.
Now, imagine trying to steer a ship when the very nature of water keeps changing. That’s what it feels like for SREs managing Generative AI systems. These aren’t the predictable, rule-based programs of the past. Instead, they’re complex, inscrutable entities capable of producing outputs as unpredictable as the weather itself.
Charting unknown waters, the challenges
The black box problem
Think of the frustration you feel when trying to understand a cryptic message from someone close to you. Multiply that by a thousand, and you’ll begin to grasp the explainability challenge in Generative AI. These models are like giant, moody teenagers, powerful, complex, and often inexplicable. Even their creators sometimes struggle to understand them. For SREs, debugging these black-box systems can feel like trying to peer into a locked room without a key.
Here, SREs face a pressing need to adopt tools and practices like ModelOps, which provide transparency and insights into the internal workings of these opaque systems. Techniques such as SHAP (Shapley Additive Explanations) and LIME (Local Interpretable Model-agnostic Explanations) are becoming increasingly important for addressing this challenge.
The fairness tightrope
Walking a tightrope while juggling flaming torches, that’s what ensuring fairness in Generative AI feels like. These models can unintentionally perpetuate or even amplify societal biases, transforming helpful tools into unintentional discriminators. SREs must be constantly vigilant, using advanced techniques to audit models for bias. Think of it like teaching a parrot to speak without picking up bad language, seemingly simple but requiring rigorous oversight.
Frameworks like AI Fairness 360 and Explainable AI are vital here, giving SREs the tools to ensure fairness is baked into the system from the start. The task isn’t just about keeping the models accurate, it’s about ensuring they remain ethical and equitable.
The hallucination problem
Imagine your GPS suddenly telling you to drive into the ocean. That’s the hallucination problem in Generative AI. These systems can occasionally produce outputs that are convincingly wrong, like a silver-tongued con artist spinning a tale. For SREs, this means ensuring systems not only stay up and running but that they don’t confidently spout nonsense.
SREs need to develop robust monitoring systems that go beyond the typical server loads and response times. They must track model outputs in real-time to catch hallucinations before they become business-critical issues. For this, leveraging advanced observability tools that monitor drift in outputs and real-time hallucination detection will be essential.
The scalability scramble
Managing Generative AI models is like trying to feed an ever-growing, always-hungry giant. Large language models, for example, are resource-hungry and demand vast computational power. The scalability challenge has pushed even the most hardened IT professionals into a constant scramble for resources.
But scalability is not just about more servers; it’s about smarter allocation of resources. Techniques like horizontal scaling, elastic cloud infrastructures, and advanced resource schedulers are critical. Furthermore, AI-optimized hardware such as TPUs (Tensor Processing Units) can help alleviate the strain, allowing SREs to keep pace with the growing demands of these AI systems.
Adapting the sails, new approaches for a new era
Monitoring in 4D
Traditional monitoring tools, which focus on basic metrics like server performance, are now inadequate, like using a compass in a magnetic storm. In this brave new world, SREs are developing advanced monitoring systems that track more than just infrastructure. Think of a control room that not only shows server loads and response times but also real-time metrics for bias drift, hallucination detection, and fairness checks.
This level of monitoring requires integrating AI-specific observability platforms like OpenTelemetry, which offer more comprehensive insights into the behavior of models in production. These tools give SREs the ability to manage the dynamic and often unpredictable nature of Generative AI.
Automation on steroids
In the past, SREs focused on automating routine tasks. Now, in the world of GenAI, automation needs to go further, it must evolve. Imagine self-healing, self-evolving systems that can detect model drift, retrain themselves, and respond to incidents before a human even notices. This is the future of SRE: infrastructure that can adapt in real time to ever-changing conditions.
Frameworks like Kubernetes and Terraform, enhanced with AI-driven orchestration, allow for this level of dynamic automation. These tools give SREs the power to maintain infrastructure with minimal human intervention, even in the face of constant change.
Testing in the Twilight Zone
Validating GenAI systems is like proofreading a book that rewrites itself every time you turn the page. SREs are developing new testing paradigms that go beyond simple input-output checks. Simulated environments are being built to stress-test models under every conceivable (and inconceivable) scenario. It’s not just about checking whether a system can add 2+2, but whether it can handle unpredictable, real-world situations.
New tools like DeepMind’s AlphaCode are pushing the boundaries of testing, creating environments where models are continuously challenged, ensuring they perform reliably across a wide range of scenarios.
The evolving SRE, part engineer, part data Scientist, all superhero
Today’s SRE is evolving at lightning speed. They’re no longer just infrastructure experts; they’re becoming part data scientist, part ethicist, and part futurist. It’s like asking a car mechanic to also be a Formula 1 driver and an environmental policy expert. Modern SREs need to understand machine learning, ethical AI deployment, and cloud infrastructure, all while keeping production systems running smoothly.
SREs are now a crucial bridge between AI researchers and the real-world deployment of AI systems. Their role demands a unique mix of skills, including the wisdom of Solomon, the patience of Job, and the problem-solving creativity of MacGyver.
Gazing into the crystal ball
As we sail into this uncharted future, one thing is clear: the role of SREs in the age of Generative AI is more critical than ever. These engineers are the guardians of our AI-powered future, ensuring that as systems become more powerful, they remain reliable, fair, and beneficial to society.
The challenges are immense, but so are the opportunities. This isn’t just about keeping websites running, it’s about managing systems that could revolutionize industries like healthcare and space exploration. SREs are at the helm, steering us toward a future where AI and human ingenuity work together in harmony.
So, the next time you chat with an AI that feels almost human, spare a thought for the SREs behind the scenes. They are the unsung heroes ensuring that our journey into the AI future is smooth, reliable, and ethical. In the age of Generative AI, SREs are not just reliability engineers, they are the navigators of our digital destiny.