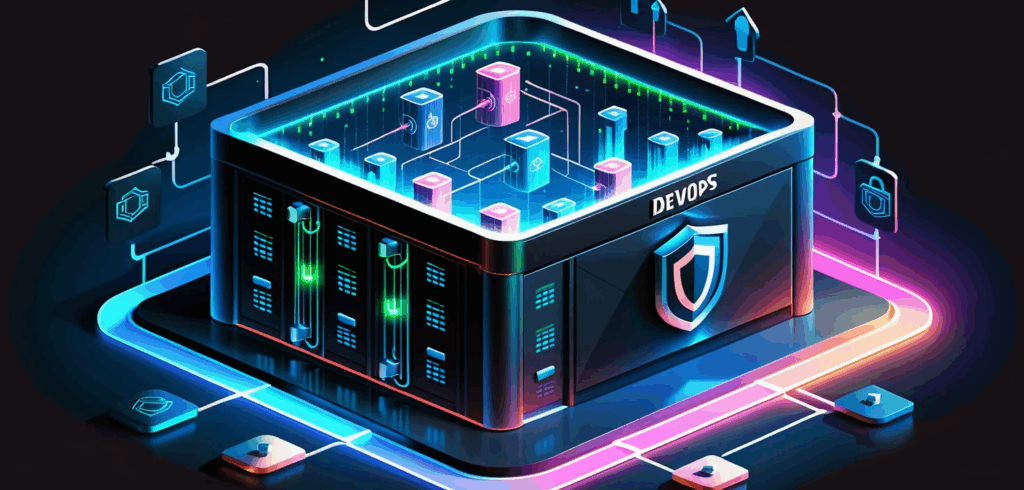
Setting up a new home isn’t merely about getting a set of keys. It’s about knowing the essentials: the location of the main water valve, the Wi-Fi password that connects you to the world, and the quirks of the thermostat that keeps you comfortable. You wouldn’t dream of scribbling your bank PIN on your debit card or leaving your front door keys conspicuously under the welcome mat. Yet, in the digital realm, many software development teams inadvertently adopt such precarious habits with their application’s critical information.
This oversight, the mismanagement of configurations and secrets, can unleash a torrent of problems: applications crashing due to incorrect settings, development cycles snarled by inconsistencies and gaping security vulnerabilities that invite disaster. But there’s a more enlightened path. Digital environments often feel like minefields; this piece explores practical strategies in DevOps for intelligent configuration and secret management, aiming to establish them as bastions of stability and security. This isn’t just about best practices; it’s about building a foundation for resilient, scalable, and secure software that lets you sleep better at night.
Configuration management, the blueprint for stability
What exactly is this “configuration” we speak of? Think of it as the unique set of instructions and adjustable parameters that dictate an application’s behavior. These are the database connection strings, the feature flags illuminating new functionalities, the API endpoints it communicates with, and the resource limits that keep it running smoothly.
Consider a chef crafting a signature dish. The core recipe remains constant, but slight adjustments to spices or ingredients can tailor it for different palates or dietary needs. Similarly, your application might run in various environments, development, testing, staging, and production. Each requires its nuanced settings. The art of configuration management is about managing these variations without rewriting the entire cookbook for every meal. It’s about having a master recipe (your codebase) and a well-organized spice rack (your externalized configurations).
The perils of digital disarray
Initially, embedding configuration settings directly into your application’s code might seem like a quick shortcut. However, this path is riddled with pitfalls that quickly escalate from minor annoyances to major operational headaches. Imagine the nightmare of deploying to production only to watch it crash and burn because a database URL was hardcoded for the staging environment. These aren’t just inconveniences; they’re potential disasters leading to:
- Deployment debacles: Promoting code across environments becomes a high-stakes gamble.
- Operational rigidity: Adapting to new requirements or scaling services turns into a monumental task.
- Security nightmares: Sensitive information, even if not a “secret,” can be inadvertently exposed.
- Consistency chaos: Different environments behave unpredictably due to divergent, hard-to-track settings.
Centralization, the tower of control
So, what’s the cornerstone of sanity in this domain? It’s an unwavering principle: separate configuration from code. But why is this separation so sacrosanct? Because it bestows upon us the power of flexibility, the gift of consistency, and a formidable shield against needless errors. By externalizing configurations, we gain:
- Environmental harmony: Tailor settings for each environment without touching a single line of code.
- Simplified updates: Modify configurations swiftly and safely.
- Enhanced security: Reduce the attack surface by keeping settings out of the codebase.
- Clear traceability: Understand what settings are active where, and when they were changed.
Meet the digital organizers, essential tools
Several powerful tools have emerged to help us master this discipline. Each offers a unique set of “superpowers”:
- HashiCorp Consul: Think of it as your application ecosystem’s central nervous system, providing service discovery and a distributed key-value store. It knows where everything is and how it should behave.
- AWS Systems Manager Parameter Store: A secure, hierarchical vault provided by AWS for your configuration data and secrets, like a meticulously organized digital filing cabinet.
- etcd: A highly reliable, distributed key-value store that often serves as the memory bank for complex systems like Kubernetes.
- Spring Cloud Config: Specifically for the Java and Spring ecosystems, it offers robust server and client-side support for externalized configuration in distributed systems, illustrating the core principles effectively.
Secrets management, guarding your digital crown jewels
Now, let’s talk about secrets. These are not just any configurations; they are the digital crown jewels of your applications. We’re referring to passwords that unlock databases, API keys that grant access to third-party services, cryptographic keys that encrypt and decrypt sensitive data, certificates that verify identity, and tokens that authorize actions.
Let’s be unequivocally clear: embedding these secrets directly into your code, even within the seemingly safe confines of a private version control repository, is akin to writing your bank account password on a postcard and mailing it. Sooner or later, unintended eyes will see it. The moment code containing a secret is cloned, branched, or backed up, that secret multiplies its chances of exposure.
The fortress approach, dedicated secret sanctuaries
Given their critical nature, secrets demand specialized handling. Generic configuration stores might not suffice. We need dedicated secret management tools, and digital fortresses designed with security as their paramount concern. These tools typically offer:
- Ironclad encryption: Secrets are encrypted both at rest (when stored) and in transit (when accessed).
- Granular access control: Precisely define who or what can access specific secrets.
- Comprehensive audit trails: Log every access attempt, successful or not, providing invaluable forensic data.
- Automated rotation: The ability to automatically change secrets regularly, minimizing the window of opportunity if a secret is compromised.
Champions of secret protection leading tools
- HashiCorp Vault: Envision this as the Fort Knox for your digital secrets, built with layers of security and fine-grained access controls that would make a dragon proud of its hoard. It’s a comprehensive solution for managing secrets across diverse environments.
- AWS Secrets Manager: Amazon’s dedicated secure vault, seamlessly integrated with other AWS services. It excels at managing, retrieving, and automatically rotating secrets like database credentials.
- Azure Key Vault: Microsoft’s offering to safeguard cryptographic keys and other secrets used by cloud applications and services within the Azure ecosystem.
- Google Cloud Secret Manager: Provides a secure and convenient way to store and manage API keys, passwords, certificates, and other sensitive data within the Google Cloud Platform.
Secure delivery, handing over the keys safely
Our configurations are neatly organized, and our secrets are locked down. But how do our applications, running in their various environments, get access to them when needed, without compromising all our hard work? This is the challenge of secure delivery. The goal is “just-in-time” access: the application receives the sensitive information precisely when it needs it, and not a moment sooner or later, and only the authorized application entity gets it.
Think of it as a highly secure courier service. The package (your secret or configuration) is only handed over to the verified recipient (your application) at the exact moment of need, and the courier (the injection mechanism) ensures no one else can peek inside or snatch it.
Common methods for this secure handover include:
- Environment variables: A widespread method where configurations and secrets are passed as variables to the application’s runtime environment. Simple, but be cautious: like a quick note passed to the application upon startup, ensure it’s not inadvertently logged or exposed in process listings.
- Volume mounts: Secrets or configuration files are securely mounted as a volume into a containerized application. The application reads them as if they were local files, but they are managed externally.
- Sidecar or Init containers (in Kubernetes/Container orchestration): Specialized helper containers run alongside your main application container. The init container might fetch secrets before the main app starts, or a sidecar might refresh them periodically, making them available through a shared local volume or network interface.
- Direct API calls: The application itself, equipped with proper credentials (like an IAM role on AWS), directly queries the configuration or secret management tool at runtime. This is a dynamic approach, ensuring the latest values are always fetched.
Wisdom in action with some practical examples
Theory is vital, but seeing these principles in action solidifies understanding. Let’s step into the shoes of a DevOps engineer for a moment. Our mission, should we choose to accept it, involves enabling our applications to securely access the information they need.
Example 1 Fetching secrets from AWS Secrets Manager with Python
Our Python application needs a database password, which is securely stored in AWS Secrets Manager. How do we achieve this feat without shouting the password across the digital rooftops?
# This Python snippet demonstrates fetching a secret from AWS Secrets Manager.
# Ensure your AWS SDK (Boto3) is configured with appropriate permissions.
import boto3
import json
# Define the secret name and AWS region
SECRET_NAME = "your_app/database_credentials" # Example secret name
REGION_NAME = "your-aws-region" # e.g., "us-east-1"
# Create a Secrets Manager client
client = boto3.client(service_name='secretsmanager', region_name=REGION_NAME)
try:
# Retrieve the secret value
get_secret_value_response = client.get_secret_value(SecretId=SECRET_NAME)
# Secrets can be stored as a string or binary.
# For a string, it's often JSON, so parse it.
if 'SecretString' in get_secret_value_response:
secret_string = get_secret_value_response['SecretString']
secret_data = json.loads(secret_string) # Assuming the secret is stored as a JSON string
db_password = secret_data.get('password') # Example key within the JSON
print("Successfully retrieved and parsed the database password.")
# Now you can use db_password to connect to your database
else:
# Handle binary secrets if necessary (less common for passwords)
# decoded_binary_secret = base64.b64decode(get_secret_value_response['SecretBinary'])
print("Secret is binary, not string. Further processing needed.")
except Exception as e:
# Robust error handling is crucial.
print(f"Error retrieving secret: {e}")
# In a real application, you'd log this and potentially have retry logic or fail gracefully.
Notice how our digital courier (the code) not only delivers the package but also reports back if there is a snag. Robust error handling isn’t just good practice; it’s essential for troubleshooting in a complex world.
Example 2 GitHub Actions tapping into HashiCorp Vault
A GitHub Actions workflow needs an API key from HashiCorp Vault to deploy an application.
# This illustrative GitHub Actions workflow snippet shows how to fetch a secret from HashiCorp Vault.
# jobs:
# deploy:
# runs-on: ubuntu-latest
# permissions: # Necessary for OIDC authentication with Vault
# id-token: write
# contents: read
# steps:
# - name: Checkout code
# uses: actions/checkout@v3
# - name: Import Secrets from HashiCorp Vault
# uses: hashicorp/vault-action@v2.7.3 # Use a specific version
# with:
# url: ${{ secrets.VAULT_ADDR }} # URL of your Vault instance, stored as a GitHub secret
# method: 'jwt' # Using JWT/OIDC authentication, common for CI/CD
# role: 'your-github-actions-role' # The role configured in Vault for GitHub Actions
# # For JWT auth, the token is automatically handled by the action using OIDC
# secrets: |
# secret/data/your_app/api_credentials api_key | MY_APP_API_KEY; # Path to secret, key in secret, desired Env Var name
# secret/data/another_service service_url | SERVICE_ENDPOINT;
# - name: Use the Secret in a deployment script
# run: |
# echo "The API key has been injected into the environment."
# # Example: ./deploy.sh --api-key "${MY_APP_API_KEY}" --service-url "${SERVICE_ENDPOINT}"
# # Or simply use the environment variable MY_APP_API_KEY directly in your script if it expects it
# if [ -z "${MY_APP_API_KEY}" ]; then
# echo "Error: API Key was not loaded!"
# exit 1
# fi
# echo "API Key is available (first 5 chars): ${MY_APP_API_KEY:0:5}..."
# echo "Service endpoint: ${SERVICE_ENDPOINT}"
# # Proceed with deployment steps that use these secrets
Here, GitHub Actions securely authenticates to Vault (perhaps using OIDC for a tokenless approach) and injects the API key as an environment variable for subsequent steps.
Example 3 Reading database URL From AWS Parameter Store with Python
An application needs its database connection URL, which is stored, perhaps as a SecureString, in the AWS Systems Manager Parameter Store.
# This Python snippet demonstrates fetching a parameter from AWS Systems Manager Parameter Store.
import boto3
# Define the parameter name and AWS region
PARAMETER_NAME = "/config/your_app/database_url" # Example parameter name
REGION_NAME = "your-aws-region" # e.g., "eu-west-1"
# Create an SSM client
client = boto3.client(service_name='ssm', region_name=REGION_NAME)
try:
# Retrieve the parameter value
# WithDecryption=True is necessary if the parameter is a SecureString
response = client.get_parameter(Name=PARAMETER_NAME, WithDecryption=True)
db_url = response['Parameter']['Value']
print(f"Successfully retrieved database URL: {db_url}")
# Now you can use db_url to configure your database connection
except Exception as e:
print(f"Error retrieving parameter: {e}")
# Implement proper logging and error handling for your application
These snippets are windows into a world of secure and automated access, drastically reducing risk.
The gold standard, essential best practices
Adopting tools is only part of the equation. True mastery comes from embracing sound principles:
- The golden rule of least privilege: Grant only the bare minimum permissions required for a task, and no more. Think of it as giving out keys that only open specific doors, not the master key to the entire digital kingdom. If an application only needs to read a specific secret, don’t give it write access or access to other secrets.
- Embrace regular secret rotation: Why this constant churning? Because even the strongest locks can be picked given enough time, or keys can be inadvertently misplaced. Regular rotation is like changing the locks periodically, ensuring that even if an old key falls into the wrong hands, it no longer opens any doors. Many secret management tools can automate this.
- Audit and monitor relentlessly: Keep meticulous records of who (or what) accessed which secrets or configurations, and when. These audit trails are invaluable for security analysis and troubleshooting.
- Maintain strict environment separation: Configurations and secrets for development, staging, and production environments must be entirely separate and distinct. Never let a development secret grant access to production resources.
- Automate with Infrastructure As Code (IaC): Define and manage your configuration stores and secret management infrastructure using code (e.g., Terraform, CloudFormation). This ensures consistency, repeatability, and version control for your security posture.
- Secure your local development loop: Developers need access to some secrets too. Don’t let this be the weak link. Use local instances of tools like Vault, or employ .env files (which are never committed to version control) managed by tools like direnv to load them into the shell.
Just as your diligent house cleaner is given keys only to the areas they need to access and not the combination to your personal safe, applications and users should operate with the minimum necessary permissions.
Forging your secure DevOps future
The journey towards robust configuration and secret management might seem daunting, but its rewards are immense. It’s the bedrock upon which secure, reliable, and efficient DevOps practices are built. This isn’t just about ticking security boxes; it’s about fostering a culture of proactive defense, operational excellence, and ultimately, developer peace of mind. Think of it like consistent maintenance for a complex machine; a little diligence upfront prevents catastrophic failures down the line.
So, this digital universe, much like that forgotten corner of your fridge, just keeps spawning new and exciting forms of… stuff. By actually mastering these fundamental principles of configuration and secret hygiene, you’re not just building less-likely-to-explode applications; you’re doing future-you a massive favor. Think of it as pre-emptive aspirin for tomorrow’s inevitable headache. Go on, take a peek at your current setup. It might feel like volunteering for digital dental work, but that sweet, sweet relief when things don’t go catastrophically wrong? Priceless. Your users will probably just keep clicking away, blissfully unaware of the chaos you’ve heroically averted. And honestly, isn’t that the quiet victory we all crave?