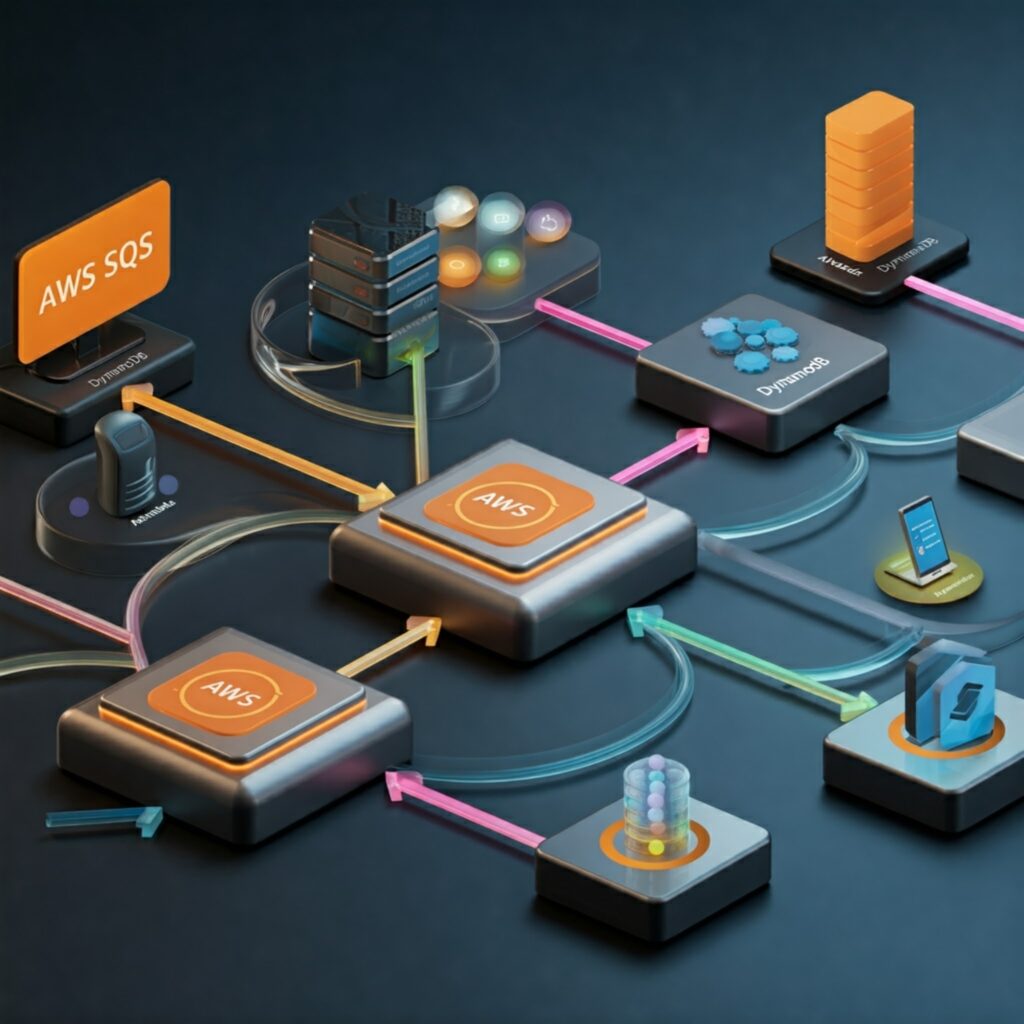
Microservices are all the rage these days, and for good reason. They offer a more flexible and scalable way to build applications compared to the old monolithic approach. However, with many independent services running around, things can get complex very quickly. This is where event-driven architecture shines, providing a robust way to manage and orchestrate microservices for better scalability, resilience, and agility.
1. Introduction
Imagine your application as a bustling city. In the past, we built applications like massive skyscrapers, and monolithic structures that housed everything in one place. But, just like cities evolve, so does software development. Modern development is more like constructing a city filled with smaller, specialized buildings that each have a specific purpose. These buildings communicate and collaborate to get things done efficiently.
This microservices approach is crucial because it allows developers to build more complex and scalable applications while remaining agile and responsive to changes. Event-driven microservices, in particular, add flexibility by enabling communication through events, allowing services to act independently and asynchronously.
2. Fundamentals of microservices architecture
2.1 Core characteristics
Think of microservices as a well-coordinated team. Each member, or service, has a specific role:
- Small, focused services: Each service is specialized, doing one thing well.
- Autonomy and loose coupling: Services operate independently and communicate through well-defined interfaces, like team members collaborating on a shared task.
- Independent data management: Each service manages its own data, ensuring data isolation and consistency.
- Team ownership: Teams take ownership of the entire lifecycle of a service, from development to deployment and maintenance.
- Resilient design: Services are designed to handle failures gracefully, preventing cascading failures and maintaining overall system stability.
2.2 Key advantages
This approach provides several benefits:
- Agile development and deployment: Smaller services are easier to develop, test, and deploy, allowing rapid iterations and responsiveness to market demands.
- Independent scalability: Each service can scale independently, optimizing resource utilization and reducing costs.
- Enhanced fault tolerance: If one service fails, the rest of the system can continue operating, ensuring high availability.
- Technological flexibility: Each service can use the most suitable technology, allowing teams to adopt the latest tools without being restricted by previous technology choices.
- Alignment with DevOps: Microservices work well with modern practices like DevOps and Continuous Integration/Continuous Delivery (CI/CD), enabling faster and more reliable releases.
3. Communication patterns in microservices
3.1 API Gateway
The API Gateway is like the central hub of our city, directing all communication traffic smoothly. It provides a single entry point for requests, manages authentication, and routes requests to the appropriate services. It also helps with cross-cutting concerns like rate limiting and caching.
3.2 Communication strategies
Microservices can communicate in various ways:
- Synchronous communication (REST/HTTP): This is like a direct phone call between services, one service makes a request to another and waits for a response. It’s straightforward but can lead to bottlenecks and dependencies.
- Asynchronous communication (Message Queues): This is akin to sending a letter, one service sends a message to a queue, and the receiver processes it at its own pace. This promotes loose coupling and improves resilience.
- Events and streaming: Like a public announcement system, one service publishes an event, and interested services subscribe and respond. This allows for real-time, scalable communication and is a key concept in event-driven architecture.
4. Event-Driven Architecture
Event-driven architecture is like a well-choreographed dance, where services react to events and trigger actions, each one moving in perfect synchrony without stepping on the toes of another. Just as dancers respond to cues, these services pick up signals and perform their designated tasks, creating a seamless flow of information and actions. This ensures that every service is aware of what it needs to do without a central authority dictating every move, allowing for flexibility and real-time responsiveness which is crucial in modern, dynamic applications.
4.1 Choreography vs Orchestration
- Choreography: Imagine a group of dancers responding to each other’s moves without a central conductor. Each dancer is attuned to the others, watching for subtle shifts in movement and adjusting their own steps accordingly. In this approach, services listen for events and react independently, much like dancers who intuitively adapt to the rhythm and flow of the music around them. There is no central authority giving instructions, yet the performance feels harmonious and coordinated. This decentralized system allows each service to be agile, responding quickly to changes without the overhead of a central controller, making it ideal for complex environments where flexibility and adaptability are key.
- Orchestration: Now picture an orchestra led by a conductor. The conductor signals each musician on when to start, how fast to play, and when to stop. In the same way, a central orchestrator manages the workflow, telling each service what to do and when. This level of centralized control can ensure that everything happens in the correct sequence, avoiding chaos and making sure all services are well synchronized. However, just like an orchestra depends heavily on the conductor, this approach introduces a potential single point of failure. If the orchestrator fails, the entire flow can come to a halt, making resilience planning critical in this setup. To mitigate this, redundancy and failover mechanisms are essential to maintain reliability.
The choice between choreography and orchestration depends on your specific needs. Choreography offers greater flexibility, allowing services to react independently and adapt quickly to changes, but it comes with less centralized control, which can make coordination challenging in more complex workflows. On the other hand, orchestration provides a high level of oversight, with a central authority ensuring all tasks happen in the right sequence. This can simplify the management of dependencies but at the cost of added complexity and potential bottlenecks. Ultimately, the decision hinges on the trade-off between autonomy and control, as well as the nature of the system’s requirements.
4.2 Event streaming
Event streaming can be thought of as a live news feed, providing a continuous stream of data that services can tap into. This enables real-time processing, allowing applications to respond to changes as they happen, such as fraud detection, personalized recommendations, or IoT analytics.
Example with AWS: Using Amazon Kinesis, you can create a streaming pipeline where data is continuously ingested, processed, and analyzed in real time. Imagine an online retail platform that needs to process user activity data, such as clicks, searches, and purchases. Amazon Kinesis acts like a real-time news broadcast where every click or search is an event being transmitted live. Different microservices listen to this data stream simultaneously. One service might update personalized recommendations based on what a user has searched for, another service might monitor suspicious activity in real-time to detect fraud, and yet another might aggregate data for business analytics, such as identifying popular products or customer behavior trends. By using Amazon Kinesis, these services can work concurrently on the same data stream, turning raw data into actionable insights immediately, much like how a news broadcast informs different departments (such as marketing, sales, and security) to take distinct actions based on the same information. This ensures that business demands are met proactively and services can adapt quickly to changing conditions.
5. Failure handling and resilience
No system is immune to failures, and that’s why effective failure-handling mechanisms are vital. Imagine a traffic signal failure in a busy city intersection, without a plan, it could lead to chaos, but with traffic officers stepping in, the flow is managed, minimizing the impact. In event-driven microservices, disruptions can lead to cascading failures if not managed correctly. Implementing robust failure handling strategies ensures that individual services can fail without bringing down the entire system, ultimately making the architecture more resilient and maintaining user trust. Designing for failure from the start helps maintain high availability, supports graceful degradation, and keeps the application responsive even under adverse conditions.
5.1 Fault tolerance strategies
- Circuit breakers: Similar to an electrical fuse, they prevent cascading failures by stopping requests to a service that is currently failing.
- Retry patterns: If a request fails, the system retries later, assuming the issue is temporary.
- Dead letter queues (DLQs): When a message can’t be processed, it is placed in a DLQ for later inspection and troubleshooting.
5.2 Idempotency
Idempotency ensures that an operation can be safely retried without adverse effects. It means that no matter how many times the same operation is performed, the outcome will always be the same, provided that the input remains unchanged. This concept is crucial in distributed systems because failures can lead to retries or repeated messages. Without idempotency, these repetitions could result in unintended consequences like duplicated records, inconsistent data states, or faulty processing.
To achieve idempotency, operations must be designed in such a way that their result remains consistent even when performed multiple times. For example, an operation that deducts from an account balance must first check if it has already processed a particular request to avoid double deductions.
This is essential for handling repeated events and ensuring consistency in distributed systems.
Example: In AWS Lambda, you can use an idempotent function to guarantee that event replays from Amazon SQS won’t alter data incorrectly. By using unique transaction IDs or checking existing state before performing actions, Lambda functions can maintain consistency and prevent unintended side effects.
6. Cloud implementation
The cloud provides an ideal platform for building event-driven microservices, offering scalability, resilience, and flexibility that traditional infrastructures often lack. AWS, in particular, has a rich ecosystem of services designed to support event-driven architectures, making it easier to deploy, manage, and scale microservices. By leveraging these cloud-native tools, developers can focus on business logic while benefiting from built-in reliability and automated scaling.
6.1 Serverless computing
Serverless computing is like renting an apartment instead of owning a house, you don’t have to worry about maintenance or management. AWS Lambda is perfect for microservices because it allows you to focus purely on the business logic without managing infrastructure. It also scales automatically with the volume of requests.
6.2 AWS services for Event-Driven microservices
AWS provides a variety of services to implement event-driven microservices:
- Amazon SQS: A message queuing service for decoupling components and handling large volumes of requests.
- Amazon SNS: A pub/sub messaging service for delivering notifications and distributing messages to multiple recipients.
- Amazon Kinesis: A real-time data streaming service for analyzing and reacting to events in real-time.
- AWS Lambda: A serverless compute service to run code in response to events, perfect for event-driven designs.
- Amazon API Gateway: A fully managed service to create and manage APIs that can trigger AWS Lambda functions.
Practical Example: Imagine an e-commerce application where a new order triggers a Lambda function via Amazon SNS. This function processes the order, updates inventory through a microservice, and sends a notification using SNS, creating a fully automated, event-driven workflow.
7. Best practices and considerations
Building successful microservices requires careful design and planning. It involves understanding both the business requirements and technical constraints to create modular, scalable, and maintainable systems. Proper planning helps in defining service boundaries, selecting appropriate communication patterns, and ensuring each microservice is resilient and independently deployable.
7.1 Design and architecture
- Optimal service size: Keep services small and focused on a single responsibility. This helps maintain simplicity and efficiency.
- Data storage patterns: Choose the right data storage solution per service, whether it’s relational databases, NoSQL, or in-memory storage, based on consistency, performance, and scalability needs.
- Versioning strategies: Use proper versioning to handle changes and maintain compatibility between services.
7.2 Operations
- Monitoring and logging: Comprehensive logging and monitoring are crucial to track performance and identify issues. Think of it as keeping an eye on every moving part of a machine. Use AWS CloudWatch Logs to collect and analyze service logs, giving you insights into how each component is behaving. Meanwhile, AWS X-Ray helps you trace requests as they move through your microservices, much like following the path of a parcel as it moves through various distribution centers. This visibility allows you to detect bottlenecks, identify performance issues, and understand system behavior in real time, enabling faster troubleshooting and optimization.
- Continuous deployment: Automate your CI/CD pipeline to deploy updates quickly and reliably. Use AWS CodePipeline in combination with Lambda to ensure new features are shipped efficiently. Continuous Deployment is about making sure that every change, once tested and verified, gets into production seamlessly. By integrating services like AWS CodeBuild, CodeDeploy, and leveraging automated testing, you create a streamlined flow from commit to deployment. This approach not only improves efficiency but also reduces human error, ensuring that your system stays up to date and can adapt to new business requirements without manual intervention.
- Configuration management: Even the best-designed cities face disruptions, which is why failure-handling mechanisms are crucial. Imagine a traffic signal failure in a busy city intersection, without a plan, it could lead to chaos, but with traffic officers stepping in, the flow is managed, and the chaos is minimized. In event-driven microservices, disruptions can lead to cascading failures if not managed correctly. Implementing robust failure handling strategies ensures that individual services can fail without bringing down the entire system, ultimately making the architecture more resilient and maintaining user trust. Designing for failure from the start helps maintain high availability, supports graceful degradation, and keeps the application responsive even under adverse conditions.
8. Final Thoughts
Event-driven microservices represent a powerful way to build scalable, resilient, and highly agile applications. By adopting AWS services, such as Lambda, SNS, and Kinesis, you can simplify the complexities of distributed systems, allowing your team to focus more on the innovations that drive value rather than the intricacies of inter-service communication.
The future of software development lies in embracing distributed architectures and event-driven designs. These approaches empower teams to decouple services, enabling each one to evolve independently while maintaining harmony across the entire system. The ability to respond to events in real time allows for dynamic, adaptable systems that can handle unpredictable workloads and changing user demands. Staying ahead of the curve means not only adopting new technologies but also adapting the mindset of continuous improvement, which ensures that your applications remain robust and competitive in the ever-changing digital landscape.
Embrace the challenge with the tools AWS provides, such as serverless capabilities and event streaming, and watch as your microservices evolve into the backbone of a truly agile, modern, and resilient application ecosystem. By leveraging these tools effectively, you’ll not only simplify operations but also unlock new possibilities for rapid scaling and enhanced fault tolerance, ultimately providing the stability and flexibility needed to thrive in today’s tech world.