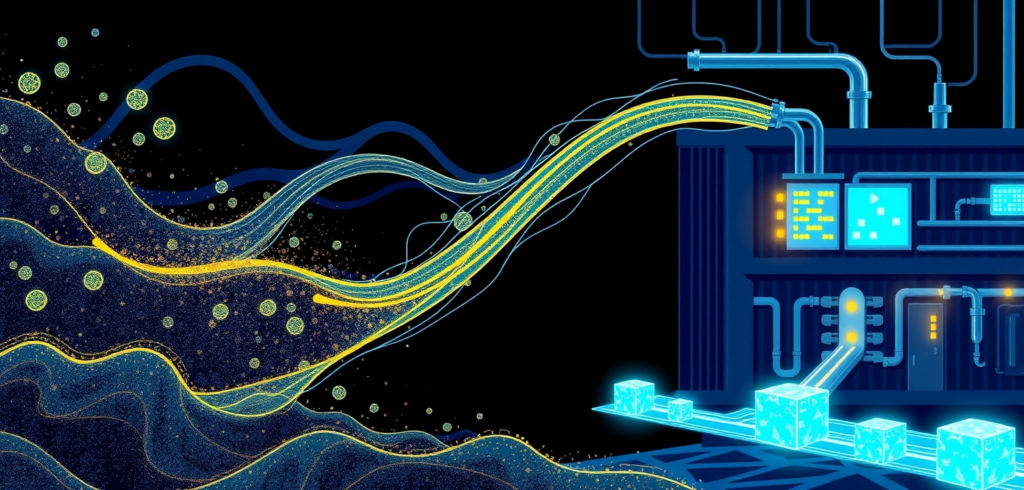
The world today runs on data. Every click, purchase, or message we send creates data, and we’re practically drowning in it. However, raw data alone isn’t helpful. Data engineering transforms this flood of information into valuable insights.
Think of data as crude oil. It is certainly valuable, but in its raw form, it’s thick, messy goo. It must be refined before it fuels anything useful. Similarly, data needs processing before it can power informed decisions. This essential refinement process is exactly what data engineering does, turning chaotic, raw data into structured, actionable information.
Without data engineering, businesses face data chaos; analysts might wait endlessly for data, or executives might make decisions blindly without reliable information. Good data engineering eliminates these issues, ensuring data flows efficiently and reliably.
Understanding what Data Engineering is
Data engineering is the hidden machinery that makes data useful for analysis. It involves building robust pipelines, efficient storage solutions, diligent data cleaning, and thorough preparation. Everything needed to move data from its source to its destination neatly and effectively.
A good data engineer is akin to a plumber laying reliable pipes, a janitor diligently cleaning up messes, and an architect ensuring the entire system remains stable and scalable. They create critical infrastructure that data scientists and analysts depend on daily.
Journey of a piece of data
Data undergoes an intriguing journey from creation to enabling insightful decisions. Let’s explore this journey step by step:
Origin of Data
Data arises everywhere, continuously and relentlessly:
- People interacting with smartphones
- Sensors operating in factories
- Transactions through online shopping
- Social media interactions
- Weather stations reporting conditions
Data arrives continuously in countless formats, structured data neatly organized in tables, free-form text, audio, images, or even streaming video.
Capturing the Data
Effectively capturing this torrent of information is critical. Data ingestion is like setting nets in a fast-flowing stream, carefully catching exactly what’s needed. Real-time data, such as stock prices, requires immediate capture, while batch data, like daily sales reports, can be handled more leisurely.
The key challenge is managing diverse data formats and varying speeds without missing crucial information.
Finding the Right Storage
Captured data requires appropriate storage, typically in three main types:
- Databases (SQL): Structured repositories for transactional data, like MySQL or PostgreSQL.
- Data Lakes: Large, flexible storage systems such as Amazon S3 or Azure Data Lake, storing raw data until it’s needed.
- Data Warehouses: Optimized for rapid analysis, combining organizational clarity and flexibility, exemplified by platforms like Snowflake, BigQuery, and Redshift.
Choosing the right storage solution depends on intended data use, volume, and accessibility requirements. Effective storage ensures data stays secure, readily accessible, and scalable.
Transforming Raw Data
Raw data often contains inaccuracies like misspelled names, incorrect date formats, duplicate records, and missing information. Data processing cleans and transforms this messy data into actionable insights. Processing might involve:
- Integrating data from multiple sources
- Computing new, derived fields
- Summarizing detailed transactions
- Normalizing currencies and units
- Extracting features for machine learning
Through careful processing, data transforms from mere potential into genuine value.
Extracting Valuable Insights
This stage brings the real payoff. Organized and clean data allows analysts to detect trends, enables data scientists to create predictive models, and helps executives accurately track business metrics. Effective data engineering streamlines this phase significantly, providing reliable and consistent results.
Ensuring Smooth Operations
Data systems aren’t “set and forget.” Pipelines can break, formats can evolve, and data volumes can surge unexpectedly. Continuous monitoring identifies issues early, while regular maintenance ensures everything runs smoothly.
Exploring Data Storage in greater detail
Let’s examine data storage options more comprehensively:
Traditional SQL Databases
Relational databases such as MySQL and PostgreSQL remain powerful because they:
- Enforce strict rules for clean data
- Easily manage complex relationships
- Ensure reliability through ACID properties (Atomicity, Consistency, Isolation, Durability)
- Provide SQL, a powerful querying language
SQL databases are perfect for transactional systems like banking or e-commerce platforms.
Versatile NoSQL Databases
NoSQL databases emerged to manage massive data volumes flexibly and scalably, with variants including:
- Document Databases (MongoDB): Ideal for semi-structured or unstructured data.
- Key-Value Stores (Redis): Perfect for quick data access and caching.
- Graph Databases (Neo4j): Excellent for data rich in relationships, like social networks.
- Column-Family Databases (Cassandra): Designed for high-volume, distributed data environments.
NoSQL databases emphasize scalability and flexibility, often compromising some consistency for better performance.
Selecting Between SQL and NoSQL
There isn’t a universally perfect choice; decisions depend on specific use cases:
- Choose SQL when data structure remains stable, consistency is critical, and relationships are complex.
- Choose NoSQL when data structures evolve quickly, scalability is paramount, or data is distributed geographically.
The CAP theorem helps balance consistency, availability, and partition tolerance to guide this decision.
Mastering the ETL process
ETL (Extract, Transform, Load) describes moving data efficiently from source systems to analytical environments:
Extract
Collect data from various sources like databases, APIs, logs, or web scrapers.
Transform
Cleanse and structure data by removing inaccuracies, standardizing formats, and eliminating duplicates.
Load
Move processed data into analytical systems, either by fully refreshing or incrementally updating.
Modern tools like Apache Airflow, NiFi, and dbt greatly enhance the efficiency and effectiveness of the ETL process.
Impact of cloud computing
Cloud computing has dramatically reshaped data engineering. Instead of maintaining costly infrastructure, businesses now rent exactly what’s needed. Cloud providers offer complete solutions for:
- Data ingestion
- Scalable storage
- Efficient processing
- Analytical warehousing
- Visualization and reporting
Cloud computing offers instant scalability, cost efficiency, and access to advanced technology, allowing engineers to focus on data challenges rather than infrastructure management. Serverless computing further simplifies this process by eliminating server-related concerns.
Essential tools for Data Engineers
Modern data engineers use several essential tools, including:
- Python: Versatile and practical for various data tasks.
- SQL: Crucial for structured data queries.
- Apache Spark: Efficiently processes large datasets.
- Apache Airflow: Effectively manages complex data pipelines.
- dbt: Incorporates software engineering best practices into data transformations.
Together, these tools form reliable and robust data systems.
The future of Data Engineering
Data engineering continues to evolve rapidly:
- Real-time data processing is becoming standard.
- DataOps encourages collaboration and automation.
- Data mesh decentralizes data ownership.
- MLOps integrates machine learning models seamlessly into production environments.
Ultimately, effective data engineering ensures reliable and efficient data flow, crucial for informed business decisions.
Summarizing
Data engineering may lack glamour, but it serves as the essential backbone of modern organizations. Without it, even the most advanced data science projects falter, resulting in misguided decisions. Reliable data engineering ensures timely and accurate data delivery, empowering analysts, data scientists, and executives alike. As businesses become increasingly data-driven, strong data engineering capabilities become not just beneficial but essential for competitive advantage and sustainable success.
In short, investing in excellent data engineering is one of the most strategic moves an organization can make.