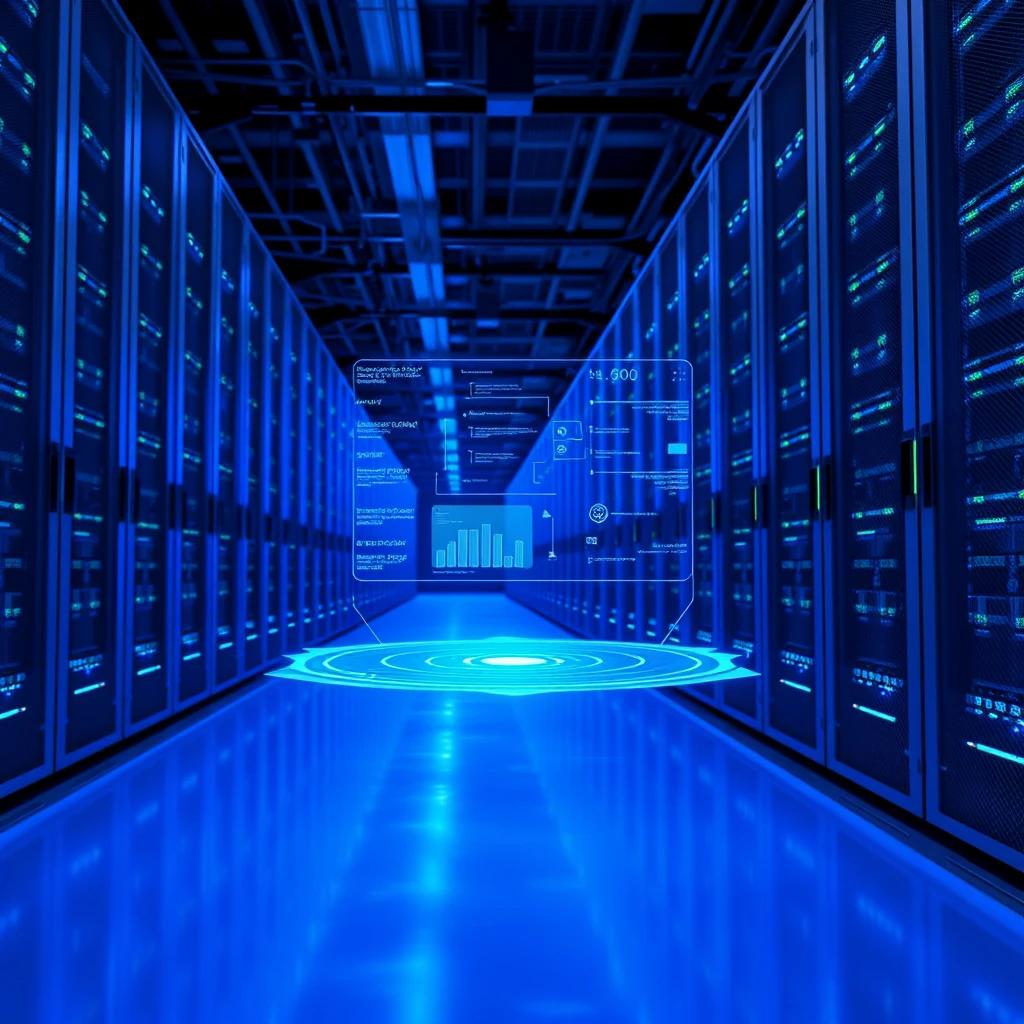
Suppose you’re conducting an orchestra where musicians can appear and disappear at will. Some charge premium rates, while others offer discounted performances but might leave mid-symphony. That’s essentially what orchestrating AWS Batch with Spot Instances feels like. Sounds intriguing. Let’s explore the mechanics of this symphony together.
What is AWS Batch, and why use it?
AWS Batch is a fully managed service that enables developers, scientists, and engineers to efficiently run hundreds, thousands, or even millions of batch computing jobs. Whether you’re processing large datasets for scientific research, rendering complex animations, or analyzing financial models, AWS Batch allows you to focus on your work. At the same time, it manages compute resources for you.
One of the most compelling features of AWS Batch is its ability to integrate seamlessly with Spot Instances, On-Demand Instances, and other AWS services like Step Functions, making it a powerful tool for scalable and cost-efficient workflows.
Optimizing costs with Spot instances
Here’s something that often gets overlooked: using Spot Instances in AWS Batch isn’t just about cost-saving, it’s about using them intelligently. Think of your job queues as sections of the orchestra. Some musicians (On-Demand instances) are reliable but costly, while others (Spot Instances) are economical but may leave during the performance.
For example, we had a data processing pipeline that was costing a fortune. By implementing a hybrid approach with AWS Batch, we slashed costs by 70%. Here’s how:
computeEnvironment:
type: MANAGED
computeResources:
type: SPOT
allocationStrategy: SPOT_CAPACITY_OPTIMIZED
instanceTypes:
- optimal
spotIoOptimizationEnabled: true
minvCpus: 0
maxvCpus: 256
The magic happens when you set up automatic failover to On-Demand instances for critical jobs:
jobQueuePriority:
spotQueue: 100
onDemandQueue: 1
jobRetryStrategy:
attempts: 2
evaluateOnExit:
- action: RETRY
onStatusReason: "Host EC2*"
This hybrid strategy ensures that your workloads are both cost-effective and resilient, making the most out of Spot Instances while safeguarding critical jobs.
Managing complex workflows with Step Functions
AWS Step Functions acts as the conductor of your data processing symphony, orchestrating workflows that use AWS Batch. It ensures that tasks are executed in parallel, retries are handled gracefully, and failures don’t derail your entire process. By visualizing workflows as state machines, Step Functions not only make it easier to design and debug processes but also offer powerful features like automatic retry policies and error handling. For example, it can orchestrate diverse tasks such as pre-processing, batch job submissions, and post-processing stages, all while monitoring execution states to ensure smooth transitions. This level of control and automation makes Step Functions an indispensable tool for managing complex, distributed workloads with AWS Batch.
Here’s a simplified pattern we’ve used repeatedly:
{
"StartAt": "ProcessBatch",
"States": {
"ProcessBatch": {
"Type": "Parallel",
"Branches": [
{
"StartAt": "ProcessDataSet1",
"States": {
"ProcessDataSet1": {
"Type": "Task",
"Resource": "arn:aws:states:::batch:submitJob",
"Parameters": {
"JobName": "ProcessDataSet1",
"JobQueue": "SpotQueue",
"JobDefinition": "DataProcessor"
},
"End": true
}
}
}
]
}
}
}
This setup scales seamlessly and keeps the workflow running smoothly, even when Spot Instances are interrupted. The resilience of Step Functions ensures that the “show” continues without missing a beat.
Achieving zero-downtime updates
One of AWS Batch’s underappreciated capabilities is performing updates without downtime. The trick? A modified blue-green deployment strategy:
- Create a new compute environment with updated configurations.
- Create a new job queue linked to both the old and new compute environments.
- Gradually shift workloads by adjusting the order of compute environments.
- Drain and delete the old environment once all jobs are complete.
Here’s an example:
aws batch create-compute-environment \
--compute-environment-name MyNewEnvironment \
--type MANAGED \
--state ENABLED \
--compute-resources file://new-compute-resources.json
aws batch create-job-queue \
--job-queue-name MyNewQueue \
--priority 100 \
--state ENABLED \
--compute-environment-order order=1,computeEnvironment=MyNewEnvironment \
order=2,computeEnvironment=MyOldEnvironment
Enhancing efficiency with multi-stage builds
Batch processing efficiency often hinges on container start-up times. We’ve seen scenarios where jobs spent more time booting up than processing data. Multi-stage builds and container reuse offer a powerful solution to this problem. By breaking down the container build process into stages, you can separate dependency installation from runtime execution, reducing redundancy and improving efficiency. Additionally, reusing pre-built containers ensures that only incremental changes are applied, which minimizes build and deployment times. This strategy not only accelerates job throughput but also optimizes resource utilization, ultimately saving costs and enhancing overall system performance.
Here’s a Dockerfile that cut our start-up times by 80%:
# Build stage
FROM python:3.9 AS builder
WORKDIR /app
COPY requirements.txt .
RUN pip install --user -r requirements.txt
# Runtime stage
FROM python:3.9-slim
WORKDIR /app
COPY --from=builder /root/.local /root/.local
COPY . .
ENV PATH=/root/.local/bin:$PATH
This approach ensures your containers are lean and quick, significantly improving job throughput.
Final thoughts
AWS Batch is like a well-conducted orchestra: its efficiency lies in the harmony of its components. By combining Spot Instances intelligently, orchestrating workflows with Step Functions, and optimizing container performance, you can build a robust, cost-effective system.
The goal isn’t just to process data, it’s to process it efficiently, reliably, and at scale. AWS Batch empowers you to handle fluctuating workloads, reduce operational overhead, and achieve significant cost savings. By leveraging the flexibility of Spot Instances, the precision of Step Functions, and the speed of optimized containers, you can transform your workflows into a seamless and scalable operation.
Think of AWS Batch as a toolbox for innovation, where each component plays a crucial role. Whether you’re handling terabytes of genomic data, simulating financial markets, or rendering complex animations, this service provides the adaptability and resilience to meet your unique needs.