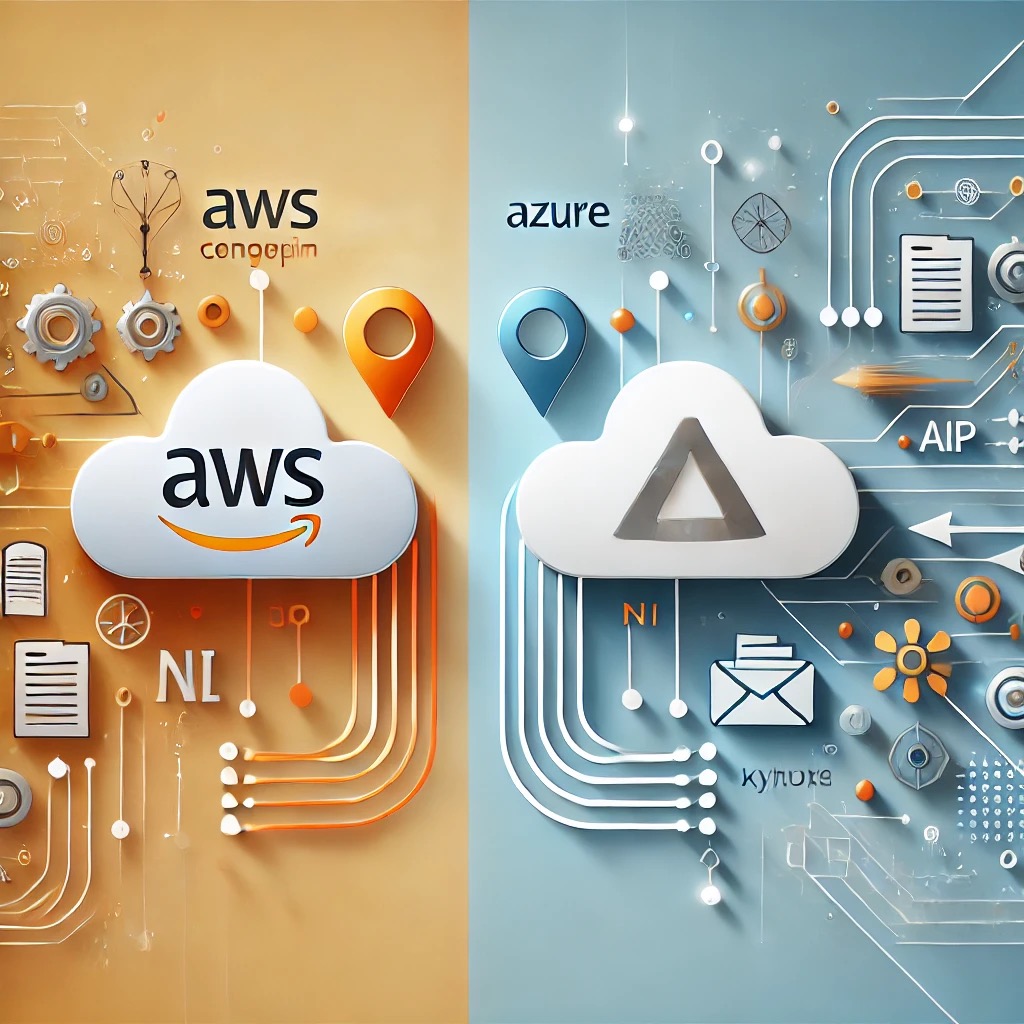
Imagine teaching a computer not only to understand human language but to grasp its subtleties, detect emotions, and reveal hidden meanings. That’s the magic of Natural Language Processing (NLP), a technology transforming industries from healthcare to finance. When you’ve interacted with customer service chatbots or received automatic insights from emails, NLP was likely behind the scenes. Today, we focus on two powerful tools driving this revolution: AWS Amazon Comprehend and Azure Text Analytics. Curious about extracting valuable insights from mountains of text? This is your starting point.
Unveiling the Titans
Let’s meet our contenders. On one side, we have AWS Amazon Comprehend, a skilled investigator meticulously sifting through text, uncovering emotions, topics, and entities. On the other side is Azure Text Analytics, a master linguist adept at breaking down language, identifying key phrases, and summarizing content. Both are packed with features, but which one should you choose? Let’s dig deeper.
AWS Amazon Comprehend. The Insightful Investigator
Think of Amazon Comprehend as a detective with a keen eye for patterns. It’s designed to dive deep into text data, revealing:
- The language of a document, even when it’s a mix of multiple languages.
- The sentiment: is the text positive, negative, or neutral?
- The main topics or themes being discussed.
- Key entities like people, places, and organizations.
- Custom models, you can train for specific tasks unique to your domain.
Imagine running an online store. Amazon Comprehend can scan customer reviews, quickly identifying whether feedback is positive or if there are issues you need to address. Or, perhaps you’re managing a news aggregator handling content in several languages. Amazon Comprehend will swiftly identify the language of each article, ensuring proper categorization and display.
Azure Text Analytics. The Language Maestro
Now, let’s turn to Azure Text Analytics, which excels at extracting critical information from large amounts of text. It can:
- Accurately identify the language of a document.
- Perform sentiment analysis, similar to Comprehend.
- Extract key phrases, the essential bits of information in a text.
- Recognize named entities like people, organizations, and locations.
- Offer custom model training to solve more specialized problems.
Picture yourself as a financial analyst swimming in endless company reports. Azure Text Analytics can summarize those documents, highlighting the essential financial figures and trends. Or, if you’re someone who likes to stay informed but lacks the time to read full articles, Text Analytics can generate concise summaries, keeping you up-to-date quickly.
Head-to-Head. Comparing the Titans
Now, let’s see how these two services compare:
Feature | AWS Comprehend | Azure Text Analytics |
Language Identification | Yes | Yes |
Sentiment Analysis | Yes | Yes |
Topic Modeling | Yes | No |
Key Phrase Extraction | No | Yes |
Named Entity Recognition | Yes | Yes |
Custom Model Training | Yes | Yes |
Pricing | Pay-as-you-go | Pay-as-you-go |
Scalability | Highly scalable | Highly scalable |
Both services are versatile, but each has its strengths. Amazon Comprehend shines when it comes to identifying hidden topics within text, while Azure Text Analytics is great for quickly pulling out key information.
Choosing Your Champion
So, which one is right for you? That depends on your specific use case. If you need to dig deep into text data and uncover hidden themes or topics, Amazon Comprehend is your go-to. However, if you’re more interested in quickly extracting key phrases or summarizing large texts, Azure Text Analytics might be your perfect match.
The best way to make an informed decision is to experiment with both. Test them with your datasets, see which one feels more intuitive, and consider the pricing to determine the most cost-effective option for your needs.
Embark on Your NLP Journey
Whether you’re a data scientist or just beginning to explore the world of NLP, both AWS Amazon Comprehend and Azure Text Analytics offer powerful tools to help you unlock the potential hidden within your text data. Don’t be afraid to roll up your sleeves and experiment with them. You might even find that they complement each other. Some projects could benefit from using both tools in different stages of analysis. The world of NLP is wide open, so dive in, explore, and start extracting valuable insights today.